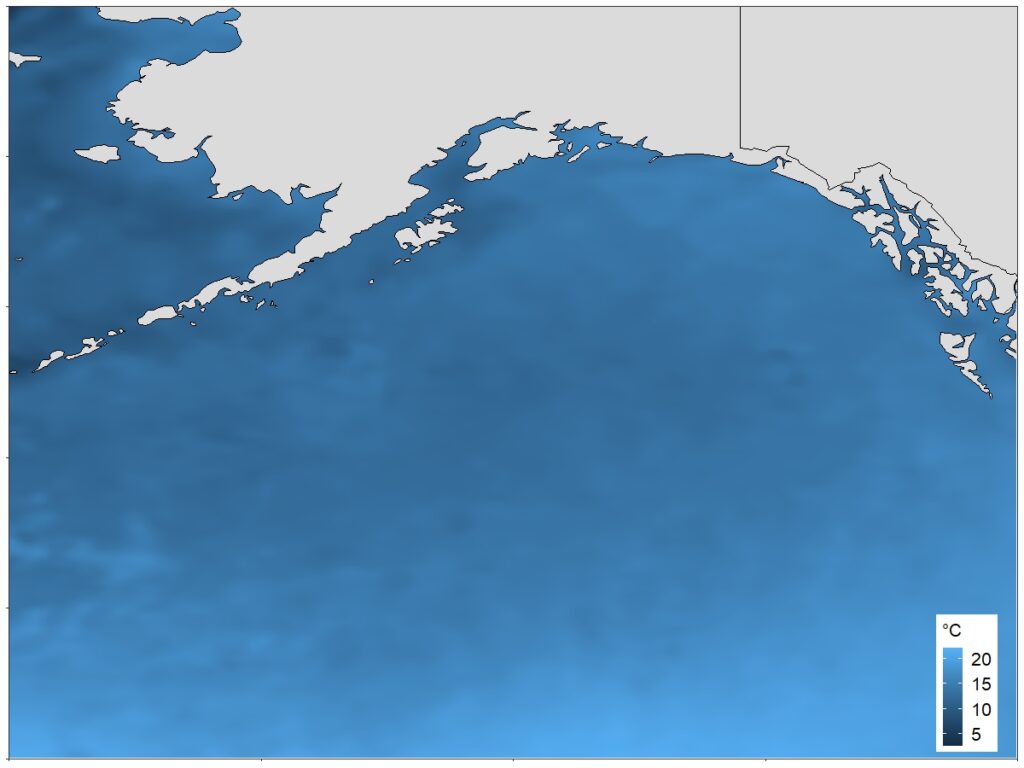
Accurate Bayesian spatial prediction using Gaussian process models
Large volumes of high-resolution geospatial data collected using remote sensing technology for scientific research present notable challenges for the commonly used Gaussian process models in spatial statistics. Assoc Prof LI Cheng‘s team studied the fixed-domain asymptotic theory from a Bayesian perspective to accurately depict the phenomenon that spatial data are collected at a higher resolution in a given region. In two papers, one published in the Annals of Statistics (December 2022) and the other forthcoming in the Journal of the American Statistical Association, the team reveals that only part of the model parameters in the Gaussian process model can be estimated accurately, while Bayesian spatial prediction at new locations remains efficient as the volume of data increases. These findings establish a solid theoretical foundation for Bayesian analysis of spatial data using Gaussian process models.
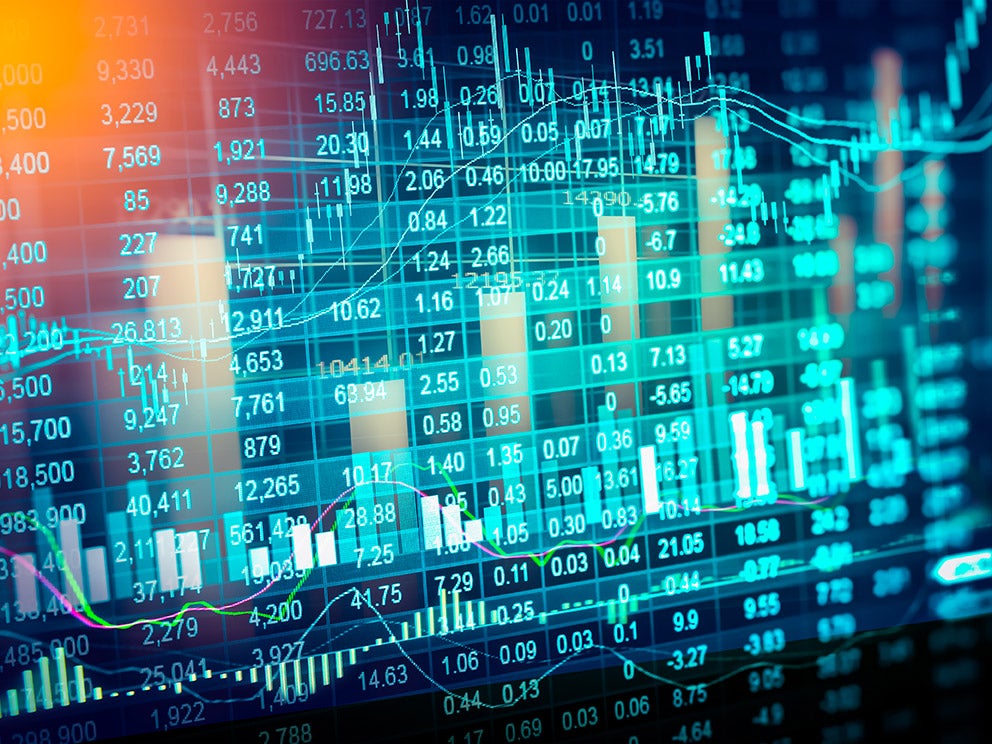
Designing efficient algorithms using data augmentation
State space models provide a unified approach for treating a diverse range of problems in time series analysis. The goal of analysis is to infer the model parameters and properties of the latent states to perform signal extraction and forecasting. State space models have prominent applications in many areas such as econometrics and ecology. Bayesian analysis of state space models has the advantage of providing uncertainty measures but is challenging due to the high dimensionality of the model. In a paper published in Statistical Science (May 2023), Asst Prof Linda TAN’s team proposed a data augmentation scheme to design efficient Markov chain Monte Carlo algorithms for Bayesian inference of some non-Gaussian and nonlinear state space models. Applications on exchange rates and trading transactions data demonstrate that the proposed methodology yields significant improvements on state-of-the-art sampling strategies.